Scientists use a variety of methods to predict mudslides, combining technology and field observations to assess risk factors.
By analyzing slope stability and integrating rainfall data, experts can forecast the conditions under which mudslides are likely to occur. Advanced predictive models take into account variables such as soil saturation and land cover, allowing for more accurate predictions.
One key element in predicting mudslides is understanding the specific terrain and vegetation in an area. Scientists employ tools like satellite imagery and ground measurements to identify regions that are prone to these events.
They can assess how heavy rainfall affects soil and rock stability, which is crucial for preventing disasters.
The increase in heavy rains due to climate change has made these predictive efforts even more important. With tools better suited for real-time analysis, researchers can issue early warnings and help communities stay safer during rainy seasons.
Fundamentals of Mudslide Prediction

Predicting mudslides involves analyzing various environmental factors, understanding the triggers that lead to these events, and leveraging advanced technologies for monitoring.
By examining these elements, scientists aim to improve predictions and mitigate risks associated with mudslides.
Understanding Mudslides
Mudslides, also known as landslides or debris flows, occur when soil and debris move down a slope due to gravity.
Key factors influencing landslide susceptibility include slope steepness, soil composition, and moisture levels.
Heavy rains often act as a significant trigger because they saturate the soil, reducing its stability. Other risk factors include past landslide activity, earthquakes, and wildfires, which can weaken the land structure. Areas with a history of shallow landslides are particularly vulnerable.
Research in geotechnical engineering helps refine models that predict how and when mudslides might occur.
Role of Climate Change and Environmental Conditions
Climate change has a direct impact on mudslide risk. Altered weather patterns lead to more intense storms and prolonged periods of heavy rainfall, increasing the likelihood of mudslides in affected regions.
Furthermore, wildfires can strip vegetation from hillsides, leaving soil exposed and more susceptible to erosion.
As a result, projects assessing landslide risk must consider these evolving climate factors.
By analyzing environmental conditions through geological surveys, scientists can develop predictive models. The data helps identify high-risk areas where preventive measures, such as constructing retaining walls or reintroducing vegetation, can be implemented.
Technological Advances in Monitoring
Recent advancements in technology have significantly improved mudslide prediction capabilities.
Tools such as LiDAR (Light Detection and Ranging) and Doppler radar are instrumental in monitoring land movements and identifying unstable areas.
LiDAR creates detailed topographic maps, allowing for precise monitoring of changes in landscapes. Early warning systems benefit from real-time data collection, enabling authorities to alert communities about potential risks.
These systems assess factors such as rainfall amounts, soil saturation levels, and surface movement.
Improved monitoring techniques offer crucial information to help mitigate the impacts of natural disasters and enhance public safety. Regular updates and assessments contribute to a more robust understanding of landslide hazards and risks.
Predictive Modeling and Early Warning Systems
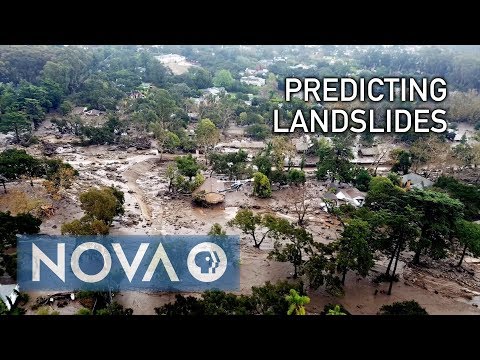
Predictive modeling and early warning systems play crucial roles in anticipating mudslide occurrences. These tools assess various factors that contribute to landslides, helping to protect communities in disaster-prone areas.
Advanced techniques, including artificial intelligence and geographical analysis, enhance the precision of these models.
Innovative Predictive Models
Researchers have developed innovative predictive models to assess mudslide risk effectively.
Deep Neural Networks (DNNs) and Superposable Neural Networks (SNNs) are at the forefront of this technology. These models analyze climatic variables, hydrology, and terrain characteristics to estimate the likelihood of landslides.
For instance, in California and the Himalayas, these models consider rainfall intensity and soil saturation.
They can predict when conditions will lead to debris flows, which often precede mudslides. The combination of machine learning and large datasets improves accuracy. This helps geologists make informed decision-making and disaster responses.
Geographic Specificity in Prediction
Geographic specificity is essential when predicting mudslides.
Models must be tailored to local conditions due to variations in climate and land use. For example, the unique geological makeup of the Italian Alps differs significantly from that of the Eastern Himalayas.
UCLA geologists focus on these nuances to refine early warning systems. They use extensive datasets to build models that consider local hydrology and rainfall patterns.
By analyzing past landslide occurrences, scientists can identify areas that may be susceptible to future events. This approach enhances risk management in specific regions.
Implementing Early Warning Strategies
Implementing effective early warning strategies is crucial for minimizing the impact of mudslides. These systems provide timely alerts based on predictive models, allowing communities to take necessary precautions.
With sophisticated technologies, such as weather monitoring and data analytics, early warnings can now offer actionable insights. Automated systems can send alerts regarding rainfall thresholds that increase mudslide risk.
Communities in high-risk areas can receive notifications to evacuate or take protective measures. For instance, combining predictive models with on-ground observations can significantly enhance safety in disaster-prone regions.
By actively monitoring conditions, timely responses can save lives and reduce property damage. By prioritizing these technological advancements, regions facing mudslide threats can better prepare for and react to potential disasters.
The integration of AI and local data helps build resilient communities against natural hazards.