Landslides are a serious natural hazard that can lead to devastating effects on communities and the environment.
The key tool used to predict landslides is a combination of predictive models and early warning systems. These systems analyze various factors such as rainfall, soil moisture, and slope stability.
These systems leverage advanced technologies, including artificial intelligence, to process vast amounts of data, improving the accuracy of landslide susceptibility assessments.
By incorporating real-time data on surface movement and weather patterns, these predictive models can provide valuable insights into when and where landslides might occur. The ability to accurately forecast these events is crucial for implementing timely safety measures.
Many experts now utilize various tools, such as remote sensing and local monitoring systems, to enhance their predictive capabilities further.
As weather patterns become more unpredictable due to climate change, understanding how these tools work is essential.
Keeping abreast of advancements in predictive modeling can help communities bolster their preparedness and response to potential landslide threats.
For more information on changes in surface movement that can signal an impending landslide, readers can explore relevant studies on surface movement analysis.
Landslide Prediction Technologies
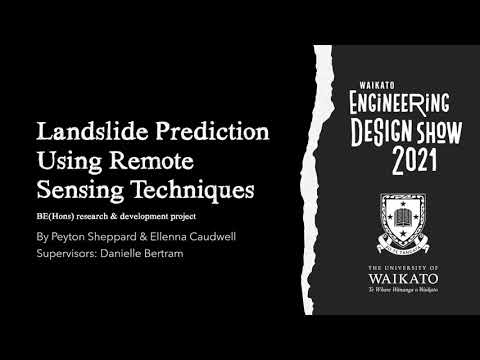
Landslide prediction relies on various technologies that enhance monitoring and forecasting efforts. These tools help identify risks and provide timely warnings to mitigate hazards associated with landslides.
Early Warning Systems and Monitoring
Early warning systems are crucial for detecting landslide risks. They use sensors placed in potential landslide areas to gather data on environmental conditions.
These systems often include inclinometers, which measure ground movement.
Remote sensing technology, like synthetic aperture radar, can track changes in land. By monitoring variables such as rainfall and soil saturation, these systems can provide real-time alerts.
Monitoring systems analyze patterns, allowing for the prediction of landslide occurrences based on conditions leading up to the event.
In some cases, these systems integrate with predictive models to improve accuracy. Such integration can effectively inform communities about potential dangers before they occur.
Artificial Intelligence and Machine Learning
Artificial intelligence (AI) and machine learning (ML) are transforming landslide prediction. Researchers use advanced algorithms, like deep neural networks, to analyze vast amounts of historical landslide data.
These ML algorithms can identify patterns that humans might miss, improving accuracy in forecasting. They analyze indicators such as rainfall intensity, soil composition, and geological features.
By employing neural networks, predictions become more reliable, allowing timely warnings.
The use of AI in landslide forecasting is increasingly common. It enables superposable neural networks to refine predictions based on new data. This approach can significantly enhance the effectiveness of early warning systems, providing a crucial edge in disaster preparedness.
Monitoring systems that utilize these technologies can lead to safer communities by ensuring that alerts reach those at risk promptly.
Assessing and Managing Landslide Risks

Assessing and managing landslide risks involves understanding various factors that contribute to hazards. This includes climate variables, land use patterns, and environmental conditions.
Effective strategies encompass hazard assessment, mapping, and integrating data into predictive models.
Hazard Assessment and Mapping
Hazard assessment is key in identifying areas susceptible to landslides. This process often includes creating a susceptibility map, which highlights regions most at risk based on geological and environmental factors.
Important contributors to susceptibility include:
- Heavy rainfall: Increased precipitation can saturate soil, making it unstable.
- Earthquakes: Seismic activity can trigger landslides in vulnerable areas.
Gathering data on historical landslide events through a landslide inventory is essential. This helps analysts understand patterns and predict future occurrences.
Engaging in comprehensive risk management means not only assessing current hazards but also considering climatic variables that may change due to climate change.
Integrating Data and Models
Integrating various data sources enhances the accuracy of landslide predictions.
Using advanced predictive models, such as statistical and physical models, helps to evaluate the likelihood of landslides under different conditions.
This integration often includes:
- Precipitation data: Monitoring moisture levels can alert authorities to increased landslide risk.
- Soil moisture readings: These provide insights into the stability of the ground.
Incorporating early warning systems is crucial for effective risk management.
By analyzing environmental conditions and integrating various data sources, models can provide timely notifications.
These proactive measures can help communities prepare for potential landslides and mitigate damage effectively.