Natural disasters can strike with little warning, leaving communities in chaos. Among various natural disasters, flash floods are often considered the hardest to predict accurately.
Despite advances in forecasting and predictive models, the sudden and intense nature of flash floods presents significant challenges for early warning systems.
Forecasting these disasters relies heavily on historical data, real-time weather conditions, and advanced risk assessment techniques.
However, the rapid changes in weather patterns can occur too fast for traditional models to respond effectively.
As a result, understanding the limitations of current prediction technology is crucial for preparing for such events.
With the frequency of flash floods increasing, it is essential to recognize the importance of enhanced predictive capabilities. Investing in better technology and improving data collection methods can lead to more effective disaster prediction, ultimately saving lives and reducing economic impacts.
Challenges in Predicting Natural Disasters

Predicting natural disasters involves various complexities. Each type of disaster presents unique challenges, making accurate forecasting difficult. Understanding these hurdles is crucial for improving preparedness and response efforts.
Earthquake Prediction Complexities
Earthquakes are among the hardest natural disasters to predict. They occur suddenly and are often caused by the sudden movement of tectonic plates.
Seismic activity can vary greatly in different areas, making it hard to use historical data for accurate predictions.
Predictive models struggle due to the lack of consistent data on smaller quakes that could indicate larger ones. Machine learning algorithms analyze past events, but the rarity of significant quakes limits their effectiveness.
Scientists often rely on limited data and cannot narrow down when or where a major earthquake might occur.
Weather-Related Disaster Forecasting
Forecasting extreme weather events like hurricanes and tornadoes uses advanced technology but still has limitations. While tools such as Doppler radar can track storms, local conditions can change rapidly, affecting forecast accuracy.
Meteorologists often face data limitations, struggling with gaps in understanding small-scale processes.
Despite advancements in machine learning and artificial intelligence, predicting sudden events like flash floods remains challenging. Historical weather patterns can inform predictions, but these models sometimes fail to capture unexpected changes in weather systems that lead to disasters.
Assessing Unpredictable Events
Some natural disasters, such as volcanic eruptions and landslides, can be particularly unpredictable. Volcanic activity might show signs, but the trigger for an eruption is not always clear.
Similarly, landslides can happen after heavy rains, but predicting the exact timing is difficult.
Droughts are another disaster that pose challenges for prediction. They develop over time, and their onset depends on numerous environmental factors.
Assessing the risk of wildfires also involves considering several variables, including moisture levels and temperature.
Technology and Advancements in Prediction Models
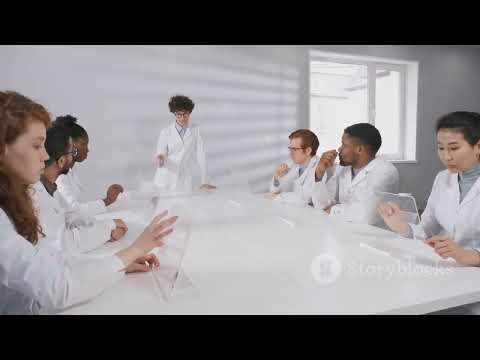
Recent advancements in technology have significantly improved the ability to predict natural disasters.
Artificial intelligence (AI) and machine learning play crucial roles in analyzing vast amounts of data. These tools help in detecting patterns linked to extreme weather events.
The National Oceanic and Atmospheric Administration (NOAA) and the National Weather Service (NWS) utilize advanced satellite data to monitor weather conditions.
This data provides real-time information about storm development and movement.
Neural networks, a form of AI, can process complex data sets. These networks analyze variables such as global temperature and atmospheric conditions.
As climate change affects weather patterns, these models become essential for accurate predictions.
Early warning systems leverage these technologies to alert emergency responders. This allows for quicker evacuations and better resource allocation during disasters.
For instance, data collected from the Internet of Things (IoT) devices can provide critical information about local conditions.
Additionally, continuous improvements in predictive analytics enable better assessment of risks.
This helps communities to prepare for potential disasters. By integrating advanced forecasting techniques, the chances of minimizing damage from extreme weather events increase.
Investing in these technologies fosters resilience against the impacts of climate change. Consequently, this prepares societies for a safer future in the face of natural disasters.