Landslides pose a significant threat to both people and infrastructure, making the ability to predict them essential.
Various technologies are used to forecast landslides, including early warning systems, remote sensing, and artificial intelligence. These methods can analyze environmental conditions that lead to landslides, allowing for timely hazard assessments and effective mitigation strategies.
Early warning systems are crucial in alerting communities about potential landslide risks.
These systems use real-time data collection tools to monitor factors such as soil moisture, rainfall, and seismic activity. When combined with advanced algorithms and machine learning models, predictions become more accurate, helping to protect lives and property.
Additionally, remote sensing technologies play a vital role in mapping areas at risk for landslides.
By utilizing satellite imagery and aerial surveys, researchers can identify danger zones and observe changes over time. This comprehensive approach enhances decision-making and preparedness in vulnerable regions, ultimately contributing to improved safety measures against landslides.
Landslide Prediction Technologies
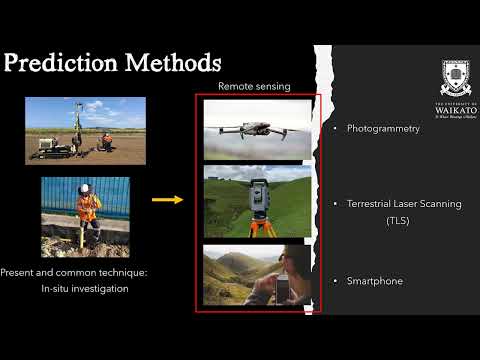
Landslide prediction involves various technologies that monitor environmental conditions and assess risks.
Key areas include remote sensing, machine learning techniques, and risk analysis frameworks that provide insights into where and why landslides may occur.
Remote Sensing and Satellite Data
Remote sensing involves collecting data about the Earth’s surface from satellites or aerial platforms.
Tools like synthetic aperture radar help detect surface movements and changes in vegetation, which are critical indicators of potential landslides. This technology enables the creation of detailed landslide maps and inventories, identifying areas susceptible to failure.
Data gathered from these methods allows for effective monitoring of climatic variables and hydrology, crucial under changing climate conditions.
By analyzing such data, researchers can inform early warning systems that alert communities at risk. Remote sensing thus plays a vital role in understanding environmental conditions that lead to landslides, allowing for timely intervention.
Machine Learning in Landslide Forecasting
Machine learning techniques, including neural networks and decision trees, enhance landslide forecasting capabilities.
These methods analyze complex data patterns to identify risk factors associated with landslides, such as rainfall and soil moisture levels. For example, deep learning models can classify regions based on landslide susceptibility, improving predictive accuracy.
Tools like the random forest and boosted regression tree algorithms are often used to create robust predictive models.
These models are instrumental in disaster-prone areas where timely predictions can save lives and protect property. As climate change intensifies, machine learning becomes increasingly crucial in adapting to shifting environmental conditions.
Risk Analysis and Hazard Assessment
Risk analysis is essential in understanding potential landslide impacts on communities.
It involves evaluating landslide risks and creating susceptibility maps that showcase areas most likely to experience landslides. This process includes detailed assessments of environmental factors, such as land use, geological features, and hydrological conditions.
Hazard assessment frameworks help in developing risk management strategies.
By integrating data from various sources, they provide actionable insights to local governments and disaster response teams. Understanding the interplay between climatic variables and landslide occurrence is vital for effective planning and community safety. Using tools like surface movement tracking within these models enhances predictive capabilities further.
Regional Case Studies and Future Outlook
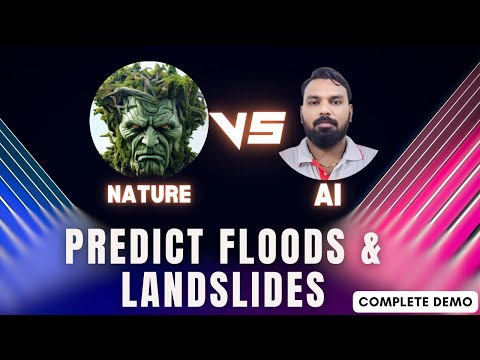
Examining specific regions sheds light on the complexities of landslide prediction and the role of environmental factors.
Two critical topics involve assessing landslide activity in the Himalayas and understanding the influence of climate change on landslide frequency.
Landslide Investigation in the Himalayas
The Himalayas are highly susceptible to landslides due to their complex geology and climatic variables. This region experiences heavy rainfall, which significantly contributes to landslide activity.
Studies emphasize landslide susceptibility mapping to identify high-risk areas and facilitate preventive measures.
Recent investigations involve analyzing risk factors such as steep slopes and earthquake history.
Researchers, including UCLA geologists, are utilizing remote sensing technologies to monitor land deformation and assess the certainty factor in predicting landslide events. These investigations provide critical data for improving warning systems and reducing vulnerabilities in communities at risk.
Climate Change and Landslide Frequency
Climate change has led to increased rainfall and temperature fluctuations, impacting landslide potential globally.
In regions like the Himalayas, these changes result in more frequent and severe natural hazards. Wildfires also contribute to changing landscapes and increase the likelihood of landslides by destabilizing soil.
Research shows a direct correlation between climatic variables and landslide occurrence.
As extreme weather events become more common, understanding these patterns is crucial for future risk assessment.
Continuous literature reviews are essential to keep track of emerging trends and improve predictive models.
Enhanced forecasting tools could play a vital role in mitigating risks associated with flooding and landslides in vulnerable areas.
For more insights on regional impacts, visit Regional – ChaseDay.com.